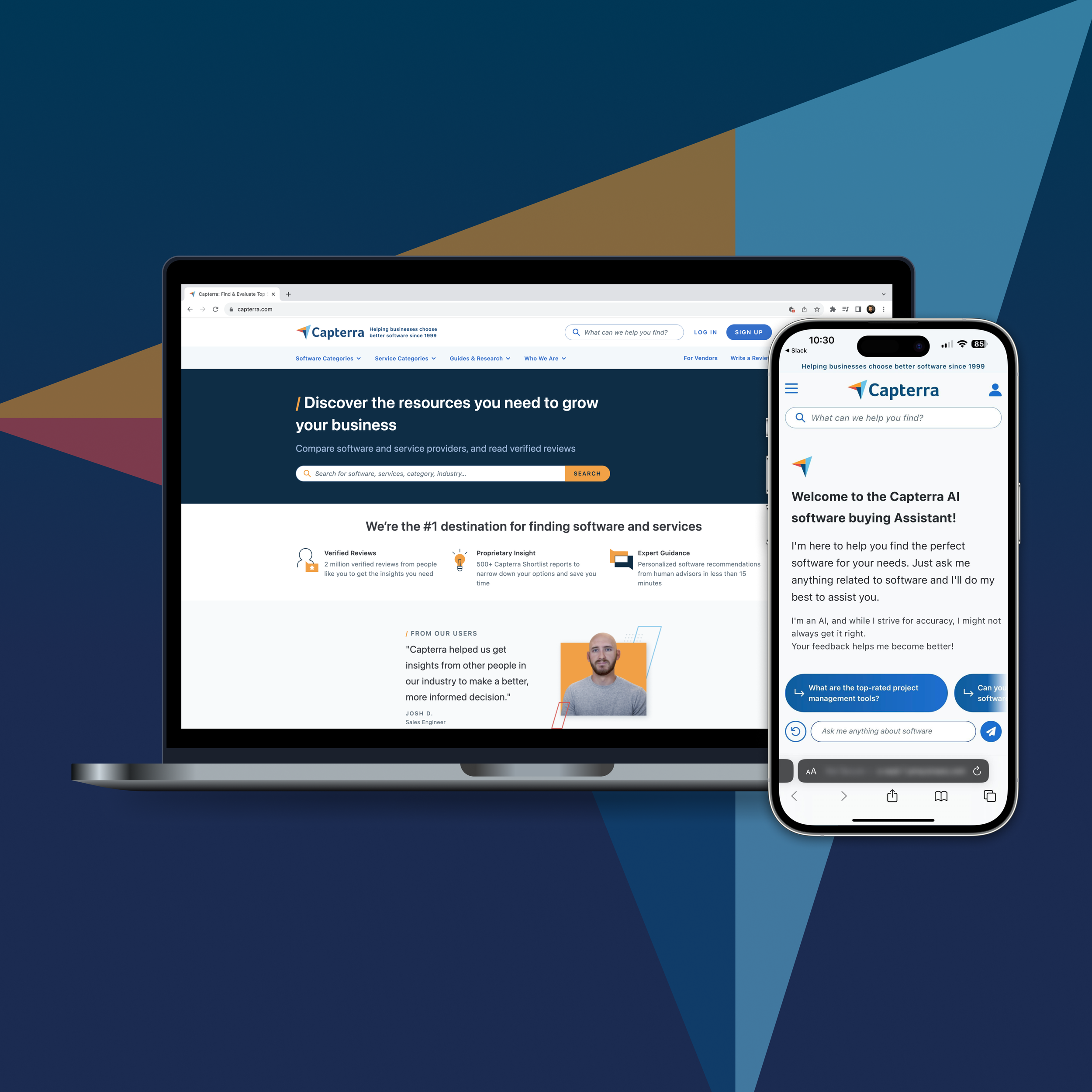
THE SOFTWARE BUYING FUTURE
How I Shaped Capterra's Generative AI Assistant
PROBLEM
Using generative AI, we wanted to create a more efficient means for software buyers to make effective decisions, ensuring an AI assistant not only provided relevant software recommendations but did so in a manner that resonated with user preferences and expectations.
The challenge was clear: in an age of information overload, how could we assist software buyers in making informed decisions efficiently? As the lead UX researcher, I was tasked with discovering user needs, pain points, and product opportunities, while guiding the project from ideation to actionable insights.
SOLUTION
Through a combination of continuous user research and competitor research, my efforts unearthed a significant user preference: the desire for visual software comparisons alongside AI-driven responses. This pivotal discovery led to design and functionality adjustments, ultimately yielding a richer, more comprehensive user experience that bridged textual and visual guidance.
In close collaboration with the product and design teams, I transformed findings into recommendations, resulting in the evolution of Capterra's AI software buying assistant. Throughout the journey, I emphasized conversation and interaction design research to ensure our AI assistant met users' expectations in every interaction.
MY RESEARCH PROCESS
PLAN
I developed a strategic research plan to align research goals with Capterra's objectives.
I created comprehensive research questions, ensuring that every aspect of a user's interaction with the AI assistant would be scrutinized and understood. I was sure to capture objectives, scope, timeline, participant information, scripts, assumptions, hypotheses, RACI roles, and related research in Coda to preserve documentation across the life of the product.
I gave stakeholders such as PMs, product designers, engineers, brand and marketing teams, and others collaborator access to this document so they could share their perspectives of the research plan.
Creating a comprehensive research plan was both invigorating and daunting. Balancing the needs of various stakeholders while keeping the user at the center was challenging. But these discussions often resulted in a richer understanding of our objectives.
Figure 1: The research plan within a Coda doc.
KICKOFF
The kickoff phase marked the onset of an energized collaborative process. Together with the multidisciplinary team, we laid out a clear roadmap:
The team swiftly designed mockups, leading to high-fidelity prototypes created in Figma.
Simultaneously, I meticulously created testing environments in UserTesting to ensure authentic user engagement and feedback.
I also established a project space in Dovetail, ensuring that the process of data capture, analysis, and synthesis was streamlined.
Throughout this phase, I maintained a continuous feedback loop with stakeholders, ensuring their insights and expectations were seamlessly integrated into the project's blueprint.
Figure 2: An early wireframe of the AI assistant.
EXECUTE
The execution phase was where the rubber met the road. Employing a combination of research methods such as prototype testing and desirability testing, I observed, surveyed, and interviewed participants using the assistant. I captured a diverse range of user interactions and feedback, painting a vivid picture of their experiences with the AI assistant.
Being hands-on during this phase reinforced the importance of adaptability in research. On a few occasions, unexpected user interactions led me to revisit and adjust our approach, ensuring we weren't just validating our assumptions but truly understanding user behavior.
Figure 3: A session recorded in UserTesting.
Figure 4: A sample of user quotes on the AI assistant.
ANALYZE
Diving deep into the data, Dovetail became instrumental as I dissected the data amassed during the execution phase.
My analytical approach, deeply rooted in Grounded Theory, guided the process. This ensured that findings emerged organically from the data, rather than being forced into preconceived notions. Through iterative coding and constant comparison, apparent patterns and themes emerged, laying a solid foundation for subsequent stages of the project.
Relying on Grounded Theory was a conscious choice. Previous experiences had shown me the pitfalls of forcing data into predefined categories. This project was a reaffirmation of the power of letting patterns emerge naturally.
Figure 5: Qualitative data analysis captured in Dovetail.
SYNTHESIZE
Taking the raw data and insights from the analysis, I distilled the information into actionable recommendations. This step was pivotal in bridging the gap between research and product development, as it transformed insights into clear user-centric designs. This phase was also a testament to the power of collaboration: seeing raw data transform into actionable recommendations reiterated the synergy between research and design.
Figure 6: Iterations informed by user research.
SHARE
I continuously acted upon an iterative design-research cycle, mirroring the principles of the double diamond approach in UX design. After gleaning findings, the team promptly refined the designs and plunged them back into the testing phase with users, ensuring each iteration was more aligned with user needs than the last.
I met frequently with the direct team to discuss these evolving insights, adapting our product strategy to consistently reflect user-centric designs. I kept stakeholders informed of developments asynchronously, granting them the freedom to engage with the data as it suited them and to seek further detail or context when needed.
Continuous iteration and sharing were more than just methodologies; they were a philosophy. By always keeping the lines of communication open, we ensured that the project was bigger than any individual—it was a collective endeavor.
Figure 7: Share-outs occurred both synchronously and asynchronously.
REFLECTION
In the journey of elevating Capterra's AI software buying assistant, not only did we achieve our project goals, but I also deepened my understanding of the intricate dance between research, design, and product. This experience reinforced the necessity of keeping users at the heart of decisions, of being adaptable in the face of new insights, and of the sheer power of collaboration.
Thank you for joining me on this journey!
If my approach resonates with you, I'd love to bring my process and more to your team.
READ MORE OF MY CASE STUDIES
Capterra’s Mobile Usability Initiative